Balancing Tech and Tradition: AI's Role in the Evolution of CAS Proceedings
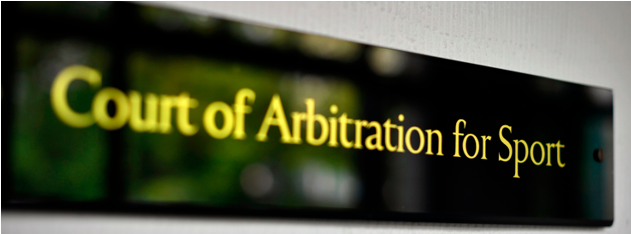
With the unprecedented advancement of data aggregation and deep learning algorithms, artificial intelligence (AI) has reshaped most practices - and sports law is no exception. In the high-stakes world of international sports, the Court of Arbitration for Sport (CAS) stands as the ultimate adjudicator of complex sporting disputes shaping athletes' careers, clubs' futures, and the integrity of global competitions, having earned its place by maintaining a standard of neutrality and expertise. Nevertheless, as proceedings continue to grow in volume and complexity, the question arises: how can CAS sustain its role while keeping pace with the demands of a digital age?
The term 'AI' refers to the process whereby large amounts of data are combined with intelligent algorithms and powerful data processing systems to enable software to automatically learn from patterns within the data.[1] As such, it presents a unique opportunity to streamline CAS proceedings, both ordinary and appeal, making arbitration faster, more efficient, and accessible to a global audience. By integrating certain AI tools, CAS can address several of its current operational shortcomings, namely: (i) the convoluted accessibility of awards, (ii)prolonged case timelines, and (iii) inconsistent awards. Thus, this article shall explore how AI can transform the general functionality of CAS in these areas and shape the future of sports dispute resolution.
To begin with, the database of CAS awards can significantly benefit from AI. Users often face limited search options and basic filtering tools that fall short when conducting in-depth research on the CAS database. The lack of cohesive categorisation and intuitive filtering options can make finding relevant cases a time-consuming and cumbersome process, potentially hindering effective case preparation. Hence, to enhance the functionality of its database, CAS can leverage specific AI tools tailored to improve search-ability, user experience, and case linkage. Firstly, an AI-powered search engine incorporating NaturalLanguage Processing (NLP) based search algorithms can select, assess, rearrange and compress information by developing algorithms that can reduce an input text to a compacted version containing just the relevant information.[2] [3] WithNLP models, CAS can summarise the information in a given award and implement more advanced search filters by date, sport, or specific legal matters, thereby enhancing the already existing ones, allowing stakeholders to quickly grasp the merits and relevant legal principles of a complex dispute, streamlining access to and research of essential case information. Furthermore, the functionality of the CAS database can significantly benefit from a machine learning-based predictive case linking. Machine learning algorithms like clustering or recommendation systems, powered by models such as collaborative filtering, can identify and suggest related cases. These systems can analyze key words and trends in previous cases to recommend relevant precedents and similar disputes, helping CAS group awards with similar merits and/or legal principles. [4] [5] This would help stakeholders discover patterns in CAS decisions and improve the transparency and consistency of case preparation.
This matter raises an additional question of the publication of awards by CAS. Although CAS publishes most awards, not all decisions are accessible to the public, significantly hindering the ability of lawyers, athletes and other stakeholders who need comprehensive access to jurisprudence. This selective publication process can thus lead to gaps or inconsistencies, inhibiting a full understanding of CAS's legal reasoning. Sometimes, it may even give one party (for example a law firm who represents many clients) an advantage in new proceedings as they have the privilege of information not available to the counter party. Whilst CAS awards have no de jure binding effect, it is widely accepted in sports arbitration that arbitrators give deference to previously established positions, essentially creating a system of de facto precedent.in this regard, any AI tool will only be as useful as the data that backs it up, and if many of CAS's awards remain unpublished it could negatively impact the ability of any AI tool. Consequently, by implementing these AI-powered summarisation, categorisation, and search functions, CAS would not only enhance the functionality behind the publication of awards, but also streamline research for legal professionals.
Secondly, AI tools can help CAS shorten procedure timelines. Ordinary and Appeals procedures inCAS typically last between 6 and 12 months. Nevertheless, depending on the complexity of the case, proceedings may extend for years despite timely submissions by parties and arbitrators. Particularly, even after arbitrators submit their respective awards, there are often significant delays in the review process, primarily due to bottlenecks at the Director-General level, where awards await final review before being officially notified. When a single individual or a small team is responsible for this crucial stage, delays become more likely, especially during high-demand periods. Given the high-stakes and time-sensitive nature of sports arbitration, where delays can disrupt careers and competition fairness, faster resolution times are crucial for maintainingCAS's credibility. Hence, to address delays in procedures and expedite the award finalisation process, CAS can utilise specific AI tools tailored for document review and workflow optimisation. On the one hand, NLP models can screen awards for consistency in formatting, citations, and procedural compliance before they reach the Director-General, reducing the number of issues requiring manual review. This would allow the Director-General's review to concentrate on substantive legal matters, expediting the overall review timeline. By identifying formatting inconsistencies, citation errors, and procedural issues, and by improving consistency in language and terminology, ensuring awards adhere to CAS standards, these tools can automate the preliminary review of awards. [6] [7] Additionally, AI workflow automation tools can help manage the flow of documents from initial drafting through to final review. These tools use machine learning to track document status, flagging drafts ready for review and prioritizing urgent cases.[8] Therefore, by automating workflow management, CAS can ensure that awards progress smoothly through each stage, reducing bottlenecks and ensuring timely handling during peak periods. In conjunction with this, machine learning models trained on CAS's case data can potentially predict peak periods and workload patterns by analyzing historical data to identify when review bottlenecks are most likely to occur, allowing CAS to anticipate and manage heavy caseloads proactively. As a result, AI tools can assist in the preliminary review process by flagging inconsistencies or procedural issues, allowing the Director-General to focus solely on substantive matters.
Finally, a significant challenge confronting CAS lies in the inconsistency in its awards, which can undermine predictability and reliability which are essential to the integrity of legal adjudication. Inconsistencies in CAS's awards seem to arise from differing interpretations of legal principles and a lack of awareness of relevant precedent among arbitrators. Thus, by integrating AI tools to run its database, CAS could support arbitrators in delivering decisions that effectively align with its established jurisprudence, promoting a much-needed cohesive approach across awards. Machine learning algorithms can examine CAS's award history to detect patterns across cases with similar facts, legal issues or principles.[9] [10] They can reveal commonalities in outcomes and applied principles, providing arbitrators with insights into how similar cases have been decided in the past. Therefore, by predicting precedents that match the specific elements of a given case, these predictive analytics tools give arbitrators a curated selection of relevant past decisions and could render a non-binding informative prompt (which ultimately does not have to be followed by an arbitrator).
To conclude, AI presents a transformative opportunity to enhance accessibility, efficiency and fairness in international sports arbitration. By implementing AI tools, CAS can tackle the selective publication and limited accessibility of awards, expedite case timelines and enhance the consistency of awards, sustaining its role as the ultimate adjudicator of international sporting disputes while keeping pace with the demands of a digital age. The improvements of each of these shortcomings would convey a thoughtful balance of tradition and innovation, with the potential to bolster the efficiency of proceedings without compromising CAS's commitment to expert and fair arbitration. Nevertheless, as AI integration continues to be explored, ongoing research and careful consideration will be essential to refine these technologies, ensuring they adequately grasp the intricacies involved in advancing the role of AI into CAS proceedings.
[1]Paisley, K. & Sussman, E. (2018). Artificial Intelligence Challenges andOpportunities for International Arbitration. NYSBA New York Dispute Resolution Lawyer, 11(1), p. 35.
[2]AL-Banna, A. & AL-Mashhadany, A. (2022). Natural Language Processing ForAutomatic text summarization [Datasets] - Survey. Wasit Journal of Computer and Mathematics Science 1(4),pp. 156-170.
[3]Suleiman, D. &, and Arafat. A. (2020). Deep learning based abstractive textsummarization: approaches, datasets, evaluation measures, and challenges. Mathematical problems in engineering 2020, 2,p. 159.
[4] Al-Omary,A. & Jamil, M. (2006). A new approach of clustering based machine-learningalgorithm. Knowledge-BasedSystems, 19(4),pp. 248-258. Retrieved from https://doi.org/10.1016/j.knosys.2005.10.011
[5] Fanca, A., Puscasiu, A.,Gota, D.I. & Valean, H. (2020). Recommendation Systems with Machine Learning, 21st International Carpathian ControlConference (ICCC), High Tatras, Slovakia, pp. 1-6.
[6] AL-Banna, A. &AL-Mashhadany, A. (2022). Natural Language Processing ForAutomatic text summarization [Datasets] - Survey. Wasit Journal of Computer and Mathematics Science 1(4),pp. 156-170.
[7]Suleiman, D. &, and Arafat. A. (2020). Deep learning based abstractive textsummarization: approaches, datasets, evaluation measures, and challenges. Mathematical problems in engineering 2020, 2,p. 159.
[8] Nandipati,S. (2021). Automating Dispute Resolution Workflows with Pega RPA and CaseManagement. Journal of Scientific andEngineering Research, 8(9), pp.300-306.
[9] Al-Omary,A. & Jamil, M. (2006). A new approach of clustering based machine-learningalgorithm. Knowledge-BasedSystems, 19(4),pp. 248-258. Retrieved from https://doi.org/10.1016/j.knosys.2005.10.011
[10] Fanca, A., Puscasiu, A.,Gota, D.I. & Valean, H. (2020). Recommendation Systems with Machine Learning, 21st International Carpathian ControlConference (ICCC), High Tatras, Slovakia, pp. 1-6.